Posted By linker 5
Posted on September 2, 2020

By H. P. Bunaes founder of AI Powered Banking.
As analytics, descriptive and predictive, are embedded in business processes in every nook and cranny of your organization, managing the operational risk associated with all of this is critical. A failure of your data analytics may, at best, impact operational efficiency, but at worst it could result in reputational damage or monetary loss. What makes this tricky is that analytics can appear to be working normally, when in fact erroneous results are being produced and sent to unsuspecting internal or external recipients downstream.
When there were only a handful of models in use, and they were developed by one group who controlled them from end to end, operational risk was manageable. But analytics is becoming pervasive, and may now be fragmented across many functions and lines of business, and operational risk is rising as a result. Many analytics groups have a long backlog of requests and resources are stretched thin. Monitoring of models in production may be low on the priority list. And, it is the rare organization indeed that knows where all the analytics in operation are and how they are being used.
Some recent examples:
● A chief analytics officer at a large US bank described how a model for approving overdrafts was found deeply embedded in the deposit system. No one remembered it was there, never mind knew how it worked.
● Another described the “what the hell” moment when data critical to credit models one day simply disappeared from the data stream.
● And a consumer banking analytics head at another bank described how models used to predict delinquencies suddenly stopped working as the pandemic hit since data used to build them was simply no longer relevant.
WHY NOW?
The topic of model risk management has been well thought through, and in some sectors, such as banking, regulatory guidance is clear. But the focus of model risk management has been on model validation and testing: all the important things that need to happen prior to implementation.
But as one head of analytics told me recently “it’s what happens after the fact that is of greatest concern [now]”. A new head of Model Risk Management at a top 10 US bank told me that “operational risk management is top of mind”. And a recently retired chief analytics officer added that unfortunately “[data scientists] just don’t get operational risk.”
In many organizations, the full extent of their deployed analytics is not known. There is no consolidated inventory of analytics, so no one knows where it all is and what it does. One large US bank last year did a survey of all of their predictive models in operation and found “thousands of models” that had not been through any formal approval, validation, or testing process according to several people I spoke with.
TOOLS AND PLATFORMS
There are tools and platforms coming on the market for managing analytics op risk (often referred to, somewhat narrowly, as “ML ops”, for machine learning operations). I’ve counted 10 of them: Verta.ai, Algorithmia, quickpath, fiddler, Domino, ModelOp, superwise.ai, DataKitchen, cnvrg.io, and DataRobot (their standalone MLops product formerly known as Parallel M). Each vendor takes a somewhat different approach to managing analytics ops risk. Over simplifying a bit, most focus either on model monitoring or on model management, only a few try to do both. Algorithmia is strong in model management, quickpath is strong in model monitoring. ModelOp and Verta.ai try to do both.
But, none of them have a prescribed operational risk management (ORM) framework. And without an effective framework for managing analytics in use, no tool will solve the problem.
In this article I will describe what an effect ORM for analytics should include at minimum.
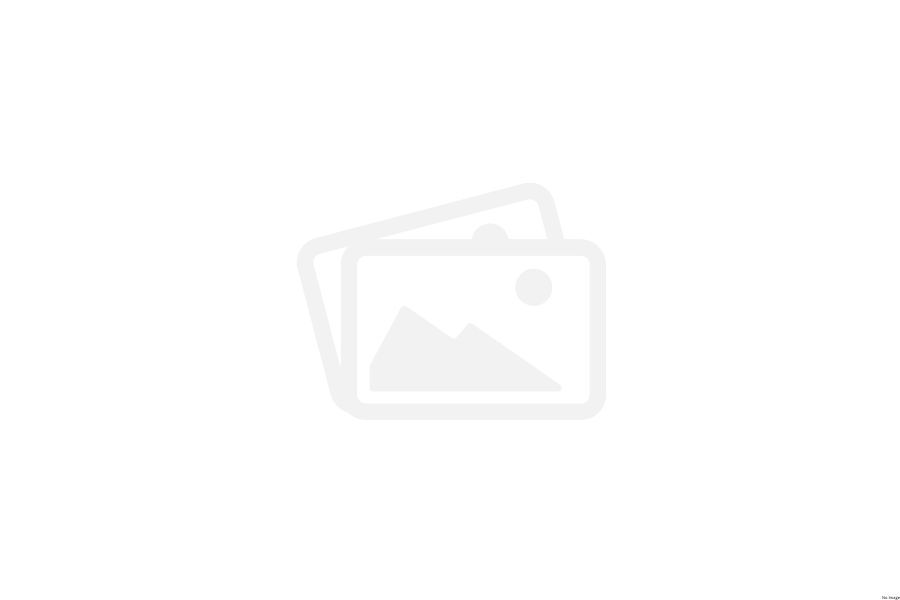
Comprehensive Operational Risk Management Framework for Analytics
MODEL MANAGEMENT
The keystone to any ORM framework is a comprehensive model inventory, a database of models including all documentation, metadata (e.g. input data used and its source and lineage, results produced and where consumed), and operational results and metrics. Knowing what and where all of your analytics are and where and how they are being used is a prerequisite for good ORM. You can’t manage what you don’t know about.
Requiring that all data about each model is captured and stored centrally prior to implementation and use is the first bit of policy I’d recommend. All of the model validation and testing done in an effective Model Risk Management process needs to be captured in the model inventory/database. And all model inputs and model outputs, their sources and their destinations need to be cataloged.
The second bit of policy is that any use of a model must be captured centrally – – who is using the model, why, and to do what? The framework falls apart if there are unknown users of models. As described in a great paper on the hidden technical debt of analytics models, a system of models can grow over time such that a change to one model can affect many downstream models. “Changing anything changes everything.”
The second critical piece to analytics operational risk management is good change management: data change management, IT change management, and model change management. Nothing ever stays the same. The environment changes, client and competitor behavior changes, upstream data sources come and go, and the IT environment is in a constant state of change. From my experience, and confirmed through many conversations with industry practitioners, the primary reason that models fail in operation is poor change management. Even subtle changes, with no obvious impact to downstream models, can have dramatic and unpredictable effects.
Changes to data need to go through a process for identifying, triaging, and remediating downstream impacts. A database of models can be used to quickly identify which models could be impacted by a change in the data. The data changes then need to be tested prior to implementation, at least for models exceeding some risk threshold. Changes to models themselves need to be tested as well when those results, even if more accurate for one purpose, are consumed by multiple applications or as inputs to other models downstream. And, of course, changes to the IT environment need to be tested to be sure that there isn’t an impact to models such as latency or performance under load.
People tend to dislike a change management process viewed as slow or bureaucratic. So change management has to be time and cost efficient. Higher priority changes going through first, for example, routine changes as a lower priority. If the change management process is slow and burdensome, people will inevitably try to go around it degrading the effectiveness of the process.
MODEL MONITORING
Model monitoring means actively watching models for signs of any degradation or of increasing risk of failure (prior to any measurable degradation). An analytics head at a top 10 US bank confided that “modelers just don’t think monitoring is important”. Monitoring must include watching the incoming data for drift, data quality problems, anomalies in the data, or combinations of data never seen before. Even subtle changes in the incoming data can have dramatic downstream effects. There must be operational metrics and logs, capturing all incoming data and outgoing results, performance relative to SLA’s, volumes over time, and a record of all control issues or process failures.
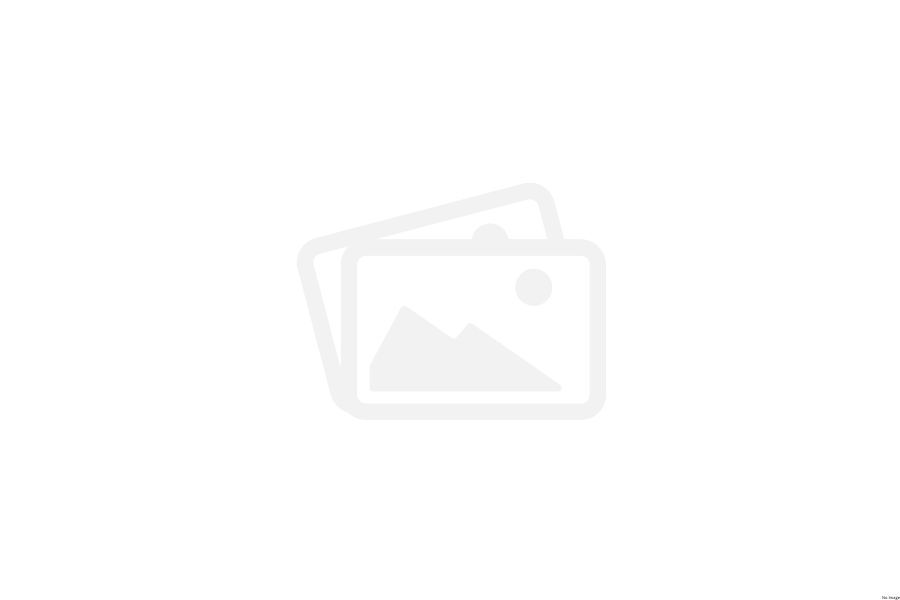
H. P. Bunaes
Operational data on models must be captured and logged to provide an audit trail, for diagnostics, and for reporting purposes. Logs should include all incoming data used in the model and all resulting predictions output, as well as volumes and latency metrics for tracking performance against SLA’s. Traceability, explainability, and reproducibility will all be necessary for 3rd line of defense auditors and regulators.
Traceability means the full data lineage from raw source data through all data preparation and manipulation steps prior to model input. Explainability means being able to show how models arrived at their predictions, including which feature values were most important to the predicted outcomes. Model reproducibility requires keeping a log not only of incoming data, but of the model version, so that results can be replicated in the future after multiple generations of changes to the data and/or the model itself.
Issue logs must be continuously updated describing any process failures (unanticipated incoming data changes), control failures (data quality problems), or outages causing models to go “off line” temporarily. Auditors and regulators will want to see a triage and escalation process, demonstrating that the big issues are identified and get the right level of attention quickly.
ETHICS AND MODEL BIAS
Models must be tested for bias and independently reviewed for fairness and appropriateness of data use. Reputational risk assessments should be completed, including a review of the use of any sensitive personal data. Models should be tested for bias across multiple demographics (gender, age, ethnicity, and location). Models used especially for decisioning such as credit approval must be independently reviewed for fairness. A record of declines, for example, should be reviewed to ensure that the model is not systematically declining any one demographic unfairly. It is an unavoidable consequence of building predictive models that any model trained on biased data will itself be biased. It may be necessary therefore to mask sensitive data from the model that could result in unintentional model bias.
REPORTING
Lastly, it is not enough to have an effective model management and monitoring process. One must be able to prove to auditors and examiners that it works. For that you need good reporting which includes:
● An inventory of all models in operation
● A log of all model changes in a specified time period (this quarter to date, last full quarter, year to date, etc): new models implemented, model upgrades, and models retrained on new data
● A log of data changes: new data introduced, new features engineered, or changes in data definitions or usage
● For changes to existing models performance metrics on out of sample test data before and after the enhancements
● For each model in production, ability to generate a detailed report of model operation including a log of data in/results out, model accuracy metrics (where absolute truth can be known after the fact), and operational metrics (number of predictions made, latency, and performance under load for operationally critical models)
● Issue log: issue description, issue priority, date of issue logging and aging, status of remediation, escalation status, actions to be taken, and individual responsible for closure, new issues and closed issues in a given period
● Operational alert history: for a given period, for each model, a report of all incoming data alerts (missing data, data errors, anomalies in the data)
● Data change management logs showing what data changed and when and which models were identified as potentially effected and tested
● IT change management logs showing changes to the infrastructure effecting models
In my experience auditors and examiners presented with a comprehensive report package for review can be satisfied that you have an effective process in place and are likely to stop there. If no such evidence is available, they will look much deeper into your organization’s use of models which will be disruptive to operations and likely result in a long list of issues for management attention.
ORGANIZATIONAL MODEL
There are multiple ways to create the right organizational partnerships for effective analytics ORM. The brute force method would be to create a new organizational unit for “analytics operations”. One could argue in favor of this approach that this new organizational unit could be built with all the right skills and expertise and could build or select the right tools and platforms to support their mission.
But a better approach might be to create a virtual organization comprised of all the key players: data scientists, data engineers (the CDO’s organization, typically), the business unit, model risk management (typically in Corporate Risk Management, but sometimes found in Finance or embedded in multiple business units), traditional IT, and audit.
Orchestrating this partnership requires clear roles and responsibilities, and well articulated and documented policies and procedures explaining the rules of the road and who’s responsible for every aspect of analytics ORM.
The latter is harder to pull off, requires more upfront thought and investment, but may yield a better and more efficient result in the long run as everyone has a stake in the success of the process and existing resources can be both leveraged and focused on the aspects of the framework they are best suited to support.
CONCLUSION
As organizations increasingly become analytics driven, a process for managing analytics operational risk will safeguard the company from unpleasant surprises and ensure that analytics continue to operate effectively. Some might argue that the process outlined here will be costly to build and operate. I would argue that (a) they are already spending more than they think on model operations, management, and maintenance (b) that unexpected failures that cascade through the data environment are always harder and more costly to fix than the cost of proactive prevention and (c) that creating a centrally managed process will free up expensive resources to do more of the high value add work the business needs. Companies that want to scale up analytics will find that an effective ORM framework creates additional capacity, speeds the process, and eliminates nasty surprises.
Author Bio:
H.P. Bunaes has 30 years experience in banking, with broad banking domain knowledge and deep expertise in data and analytics. After retiring from banking H.P. led the financial services industry vertical at DataRobot, designing the Go To Market strategy for banking and fintech, and advising 100’s of banks and fintechs on data and analytics strategy. H.P. recently founded AI Powered Banking (https://aipoweredbanking.net) with a mission of helping banks and fintechs leverage their data and advanced analytics and helping technology firms craft their GTM strategy for the financial services sector. H.P. is a graduate of M.I.T. where he earned an M.S. in Information Technology.
This is a Sponsored Feature.